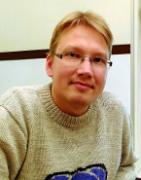
Writing a User-defined datatype
Walk-through of extending PostgreSQL with a user-defined type. The journey begins from the basics, from creating simple domain types over existing types, and continues to implementing a full-blown datatype from scratch in C.
PostgreSQL's advanced index types, GiST, GIN, and SP-GiST, are covered in enough detail to give an understanding of what each of them is good for. Support functions for each of them are shown for the example 'color' datatype.
Слайды
Видео
Другие доклады
-
Игорь Косенков Postgres Professional Администратор БД
Развертывание отказоустойчивого кластера Postgres на pacemaker
Если вы давно присматриваетесь к отказоустойчивым решениям, то наверняка слышали про кластеры СУБД на основе Corosync&Pacemaker.
По видам эти кластеры можно разделить на 3-х узловой и 2-х узловой с голосующим узлом. По размещению кластеры делятся на кластеры на физических серверах и в виртуальной среде. В чем их отличия и особенности их настройки?
Об этом вы узнаете, посетив мой мастер-класс. Вы также убедитесь, что установка и настройка кластера на Pacemaker не так сложна и трудоемка, как может показаться на первый взгляд.
-
Shawn Kim Apposha CEO
Make Your PostgreSQL 10x Faster on Cloud in Minutes
Cloud storage has some unique characteristics compared to traditional storage mainly because it is virtualized and controlled by software. One example is that AWS EBS shows higher throughput with larger I/O size up to 256 KiB without hurting latency. Hence, a user can get only about 4 MiB/sec with 1,000 IOPS EBS volume if the I/O request size is 4 KiB, whereas a user can get about 250 MiB/sec if the I/O request size is 256 KiB. This is because EBS consumes one I/O in a given IOPS budget for every I/O request regardless of the I/O size (up to 256 KiB). Unfortunately, PostgreSQL cannot exploit the full potential of cloud storage because PostgreSQL has designed without considering the unique characteristics of cloud storage.
In this talk, I will introduce the AppOS extension that improves the throughput of a write-intensive workload by 10x by transparently making PostgreSQL cloud storage-native. AppOS works like a storage driver that efficiently exploits the characteristics of cloud storage, such as I/O size dependency to storage throughput and latency, atomic write support in cloud block storage, and fast, but non-durable local SSDs. To do this, AppOS comprises a Linux-compatible file I/O stack including virtual file system, page cache, block I/O layer, cloud storage driver. On top of the file I/O stack, syscall module supports registering pre- and post-handler for file I/O-related system calls in order to transparently work without modifying PostgreSQL codes.
I will focus on presenting key use cases and performance results of the AppOS extension after explaining the internals. Specifically, I will show the performance results of OLTP and some batch workloads using standard benchmarking tools like pgbench and sysbench. I will also present performance results and implications on multiple clouds including AWS, GCP, and Azure.
-
Брюс Момжиан EnterpriseDB Senior Database Architect
Unlocking the Postgres Lock Manager
Locking is critical for providing high concurrency for any database — you cannot fully utilize your hardware if locking is throttling its use. This talk explores all aspects of locking in Postgres by showing queries and their locks; covered lock types include row, table, shared, exclusive, and advisory lock types. The high concurrency provided by Multiversion Concurrency Control (MVCC) is also covered.
Slides are at https://momjian.us/main/writings/pgsql/locking.pdf
-
Christopher Travers DeliveryHero SE Principle Engineer
Introducing Bagger: Massive Application Log Management on PostgreSQL
This talk discusses the open source components we use at Adjust to manage a massive number (5+PB) of application log messages on PostgreSQL in a massively multi-parallel way. It provides both a use case for PostgreSQL in a big data (high volume/velocity/variety) environment, and can be used to show the power of PostgreSQL with JSONB, GIN, and more.
This talk covers the capabilities of the components in depth, sufficient to inspire similar solutions.