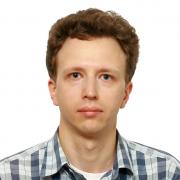
Dmitry Melnik
ISP RAS
14:00
17 March
22 min
Dynamic Compilation of SQL Queries in PostgreSQL Using LLVM JIT
Currently, to execute SQL queries PostgreSQL uses interpreter, which implements Volcano-style iteration model. At the same time it’s possible to get significant speedup by dynamically JIT-compiling query “on-the-fly”. In this case it’s possible to generate code that is specialized for given SQL query, and perform compiler optimizations using the information about table structure and data types that is already known at run time. This approach is especially important for complex queries, which performance is CPU-bound.