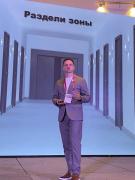
Anton Doroshkevich
InfoSoft
10:15
25 October
45 min
Unequal battle with "bloating" in the realities of 1C databases
Data is getting bigger, disks are getting faster, the DBMS optimizer is getting smarter, but the problem of " bloating " remains extremely relevant. I want to share my experience and approach to dealing with this effect on a large number of tables and data in them.