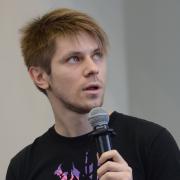
Towards more efficient query plans: PostgreSQL 11 and beyond
A major responsibility of a database engine is to convert a declarative SQL query to an efficient execution plan, employing various methods to scan and join the relations. There is always a development effort to improve this area. What clever execution plans can PostgreSQL generate, what's new in version 11 and what is in development? To name a few things, the joins are optimized by removing unneeded outer and inner joins, and reducing joins from outer and semi to inner. There is work to enable merge joins on inequality and range overlap, and to improve join selectivity estimates with multi-column statistics. When it comes to scanning a single relation, covering indexes allow to use index-only scans more often. Incremental sort and more precise estimation of sorting costs help generate better paths when sorted output is required, e.g. when using GROUP BY and ORDER BY or performing merge joins. This talk aims to give an overview of such optimizations that already exist and that are being developed now.