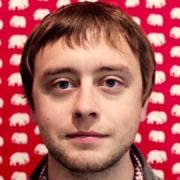
Will Leinweber
Heroku
11:15
05 February
Heroku Postgres: architecture of a cloud database service
In addition to providing a general purpose web platform, Heroku has a large, supporting Postgres service. Over the years, we've learned a lot about running Postgres at scale.
In this talk, we'll cover:
- why Postgres is attractive to run as a cloud service
- how to provision, manage, and monitor a Postgres fleet
- tradeoffs needed to make Postgres work in this environment
- automating failure recovery
- and more