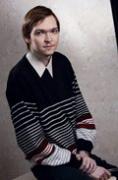
Kirill Borovikov
10:00
05 February
45 min
Plan + query = ?.. Finding pleasure in analyzing query plans
Odd things in query plan analysis - wasted time and "unnecessary" buffers.
Structural hints in a plan. How to help a developer with optimization without writing a single line of code. How to match plan nodes with query text and take advantage of this information.