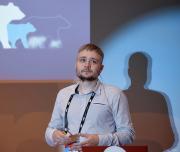
Ivan Muratov
First Monitorung Company LLC
17:45
25 October
45 min
TimescaleDB 2.0 - Time-series data in TimescaleDB distributed cluster on top of PostgreSQL ORDBMS.
TimescaleDB extension allows to turn good old Postgres into a real distributed cluster for storing time series data while maintaining the relational model, convenient SQL and a time-tested ecosystem. And additional features such as continuous materialized views and data compression allow to build truly powerful telematic hubs.