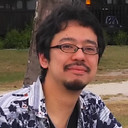
Updating Materialized Views Automatically and Incrementally
Materialized view is a feature to store the results of view definition queries in DB in order to achieve faster query response. However, the data in the view gets stale after underlying tables are modified. Therefore, view maintenance is needed to keep the contents up to date. PostgreSQL has REFRESH MATERIALIZED VIEW command for updating a materialized view, but this command needs to recompute the contents from scratch, so this is not efficient in cases where only a small part of a base table is modified.
Incremental View Maintenance (IVM) is a technique to maintain materialized views efficiently, which computes and applies only the incremental changes to the materialized views instead of recomputing. This feature is required for updating materialized views rapidly but not implemented on PostgreSQL yet.
Therefore, we developed IVM on PostgreSQL and are proposing to implement this as a core feature. The patch is now under discussion on the hackers mailing list. Our implementation allows materialized views to be updated automatically and incrementally when a underlying table is modified. You don't need to write your own trigger function for updating views. As a result of continuous development, the current implementation supports some aggregates, subqueries, self-join, outer joins, and CTEs (WITH clauses) in a view definition query. The result of performance evaluation using TPC-H queries shows that our IVM implementation can update a materialized view more than 200 times faster than re-computation by REFRESH command.
In this talk, we will describe our IVM implementation and its features.
Видео
Другие доклады
-
Mikhail Tsvetkov Intel
Intel Technologies for PostgreSQL
In this presentation, we'll discuss Intel products and solutions intended for the Data Platform Group segment, such as Xeon 3rd Gen (4S Cooper Lake) server CPUs, PMEm 200 Series RAM and FPGA.
-
Dmitry Dolgov Zalando SE
How many engineers does it take to make subscripting work?
Recently landed in PostgreSQL, jsonb subscripting support doesn't look as exciting as some other improvements around jsonb. But it's user visible changes are only tip of the iceberg. How many people were involved to make it, and what decisions choices were made? How long did it take, and what are the good/bad ideas to promote a patch? These and few other questions will be our targets in this talk.
-
Arseniy Sher Postgres Professional
Consensus, Postgres, Multimaster
Postgres Pro Multimaster is Postgres extension (and a set of core patches) providing high availability (HA) with strong consistency and read scalability. It forms symmetric shared-nothing cluster synchronously replicating the data and automatically performing disaster recovery. During the last year we've put significant efforts ensuring and proving that consistency is preserved in all scenarios. The new version, which will be released as part of Postgres Pro Enterprise 13 uses Paxos algorithm for determining transaction outcome and custom protocol governing the recovery process; we used TLA+ model checker to verify its correctness. I'll tell how these things work and why in some cases multimaster may be an attractive alternative to the traditional streaming replication based HA deployments.
multimaster is now open source, available at https://github.com/postgrespro/mmts
To make the talk less narrow specialized and more appealing to the wide audience, in the first part I will shed some light on how generally modern DBMSs (mostly so-called NewSQL) handle fault tolerance. In particular,
- what is a strongly consistent DBMS and the associated overhead;
- what is distributed consensus, Paxos, Raft;
- how they help here;
I won't do an attempt to explain any algorithms line-by-line; it would be hardly useful given the time frames and there is a lot of literature available anyway. The goal is rather to waymark the field and get you a bit comfortable there.
-
Anton Doroshkevich ИнфоСофт
Postgres Pro Data Compression (CFS) for 1C ERP platform data
Postgres Pro Enterprise has a great compression engine. The year 2020 was devoted to the study of this mechanism in the real work of 1C. We have accumulated some statistical data and of course the subtleties of the use and behavior of 1C compared to other popular DBMS, which I want to share.