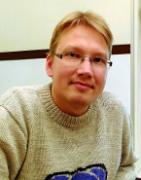
Heikki Linnakangas
Pivotal
PostgreSQL hacker
17:30
05 февраля
45 мин
Writing a User-defined datatype
Walk-through of extending PostgreSQL with a user-defined type. The journey begins from the basics, from creating simple domain types over existing types, and continues to implementing a full-blown datatype from scratch in C.
PostgreSQL's advanced index types, GiST, GIN, and SP-GiST, are covered in enough detail to give an understanding of what each of them is good for. Support functions for each of them are shown for the example 'color' datatype.